Why the 2020 census has 9 fake people in a single house
The Census Bureau added "noise" to its data to protect respondent privacy.
Last week I set out to visit a particular house about four miles north of the White House. Thanks to an unusual confluence of streets, it sat by itself on a tiny, triangular block.
That made it an easy way to check the work of the Census Bureau. The agency has published data from the 2020 Census down to the level of individual blocks, most of which have dozens of homes. This block had only one.
According to census data, this block—and hence this house—had 14 residents in 2020: one Hispanic person, seven white people, three biracial people (white and black), two multi-racial people (white, black, and American Indian), and one person of “some other race.” There were supposedly eight adults and six children living in the house.
I wanted to find out if these figures were accurate, but a sign on the gate said “no soliciting.” So I left a note asking the homeowner to call me. A few hours later, she did. She told me that her household currently has five people—and didn’t have more than that in 2020.
“We’re all just white people,” she added with a chuckle.
When I asked a Census Bureau spokeswoman about this, she pointed me to the agency’s website. A document there describes how the agency randomly adds fake people to some blocks and subtracts real people from others. The goal of this odd-sounding exercise is to protect Americans’ privacy by making it difficult to guess any individual’s response to the 2020 Census.
To be fair to the Census Bureau, a one-home block is the worst-case scenario for this privacy scheme. In percentage terms, the agency adds the most “noise” to the smallest blocks. This block-level statistical noise is designed to largely cancel out as data is aggregated into larger geographical units.
Still, block-level data for 2020 will be less accurate than it was for 2010. And that will make it harder—perhaps even impossible—to do certain types of research. This has triggered a backlash among users of census data who argue that the more limited privacy measures taken in 2010 worked fine.
“I don't even understand the threat,” said University of Arizona legal scholar Jane Bambauer in a phone interview. To her, the new privacy protections seem like a solution in search of a problem.
One reason social scientists are up in arms about this is that the Census Bureau is planning to extend the new privacy approach to surveys and datasets beyond the 2020 Census. That includes the widely used American Community Survey, which asks Americans about everything from their incomes to their commuting patterns. Because the ACS includes anonymized data about individuals—microdata, in statistics jargon—it will be difficult to release in a way that satisfies the Census Bureau’s new, more stringent privacy standards. This means the ACS could soon become a lot less accurate, and hence a lot less useful to researchers and policymakers trying to understand the American economy.
The specter of re-identification attacks
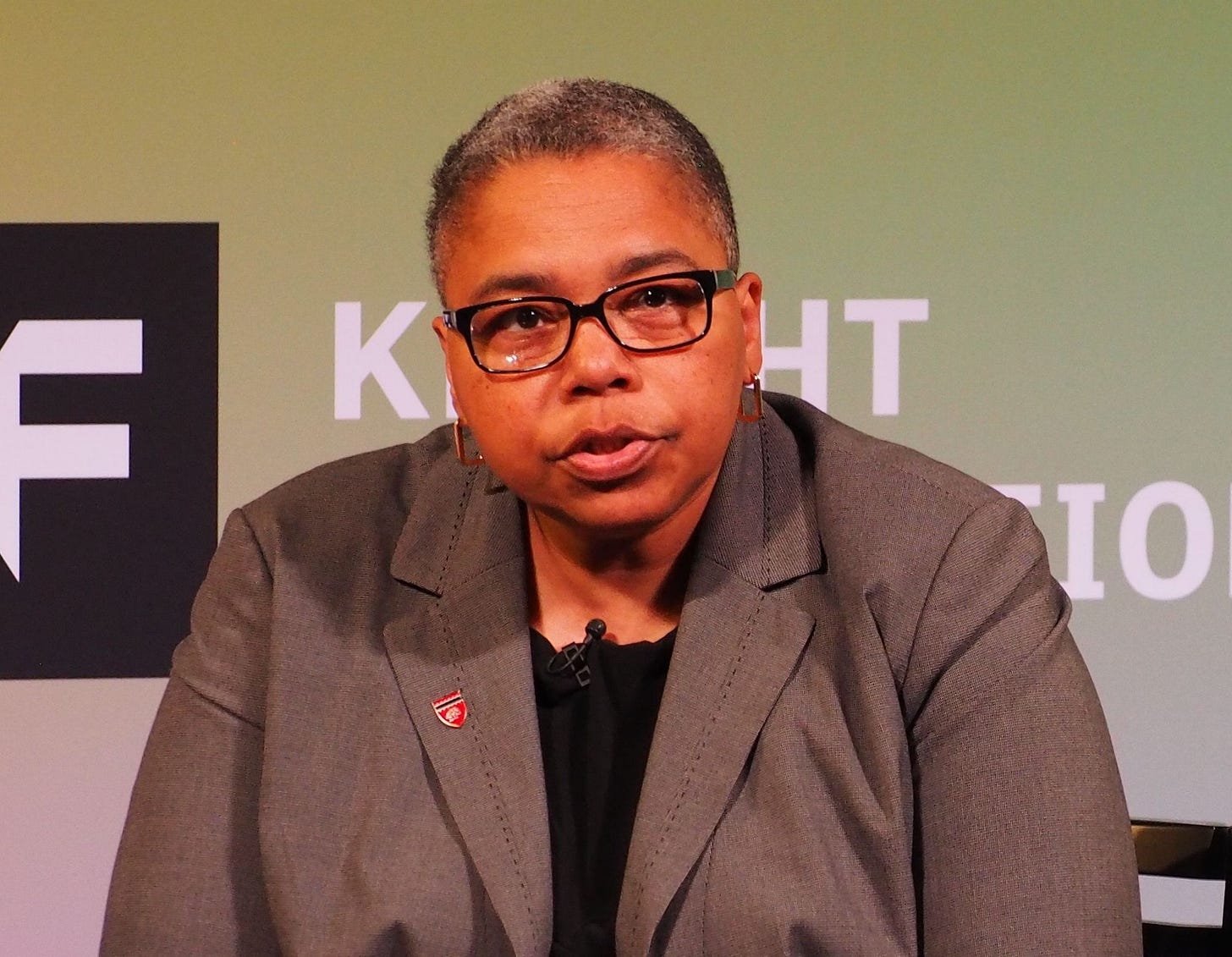
The Census Bureau’s new approach is rooted in computer science research showing that it can be surprisingly easy to unmask individuals in supposedly anonymous data sets.
In the 1990s, computer scientist Latanya Sweeney figured out how to identify individuals in a supposedly anonymized data set of Massachusetts hospital records. In a theatrical flourish, she figured out which records belonged to then-governor William Weld and sent them to his office.
Then in 2006, computer scientist Arvind Narayanan demonstrated he could identify individuals in a supposedly anonymized database of private Netflix ratings. While most movie ratings are innocuous, Narayanan pointed out that some could offer clues about sensitive topics like a user’s political and religious views or sexual orientation.
“What happened in the computer science community over time is that people became more and more suspicious of intuitive arguments for the privacy of statistical data sets,” computer scientist Ed Felten told me in a February interview.
Computer scientists also started to worry about the privacy implications of publishing summary statistics about groups of individuals.
If I tell you the average height of five people is 69 inches, that doesn’t reveal the height of any individual. But suppose I also tell you there are four men and one woman, and that the men have an average height of 70 inches. Then the woman’s height must be 65 inches.
This is known as a database reconstruction attack, and it can be done on a much larger scale than this toy example. The more summary statistics published about a dataset, the more potential there is to reverse-engineer the individual data points.
The Census Bureau has published billions of statistics derived from the 2010 census. A few years ago, agency officials started to worry that someone with vast computing power and sophisticated algorithms might be able to reveal the answers millions of individuals gave on census forms. That would be a big problem because the confidentiality of census records is protected by law.
“People are pretty damn safe”—or are they?
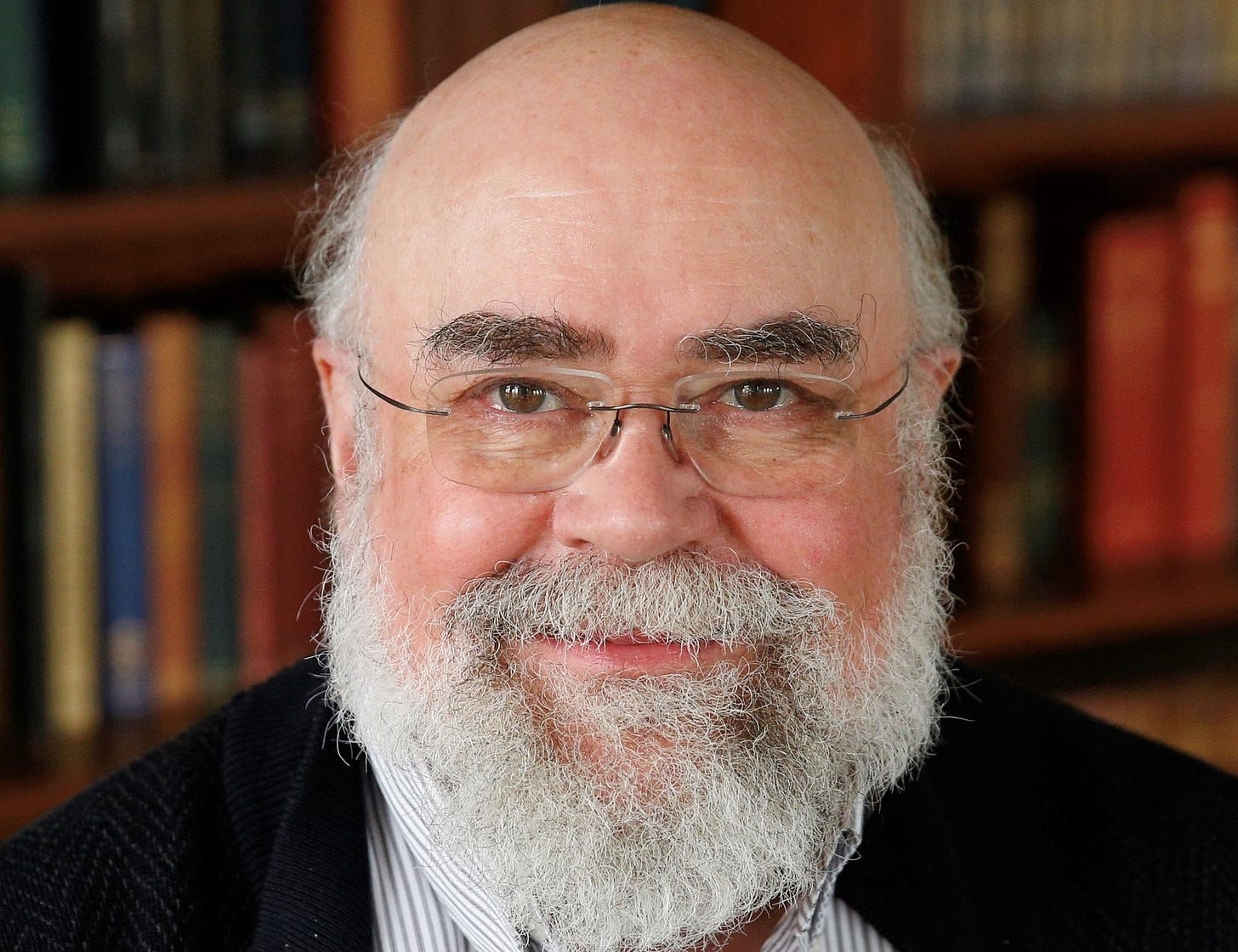
Let’s think again about the house I mentioned at the start of the article. The Census Bureau reported that it had 14 residents in 2020 when it actually had five or fewer. That’s a big, rather clumsy change to the data to safeguard the privacy of the respondents.
In 2010, the agency would have taken a less drastic approach. Steven Ruggles, a demographer and historian at the University of Minnesota, explained the old privacy method to me in a recent video call.
“When there's tiny blocks like that they swap them with someone from a nearby block with generally similar characteristics,” Ruggles said.
The agency didn’t necessarily swap everyone on a small block—just enough to create uncertainty about what anyone actually wrote on their census form.
Many social scientists prefer this old approach because it was less distortionary. Swapping left the number of people in a block unchanged, and it only slightly disturbed the distribution of people by age and race.
And folks like Ruggles argue the old method was sufficient to protect the confidentiality of census responses. “With swapping, people are pretty damn safe,” Ruggles told me.
But privacy advocates worried that even with data swapping, someone might be able to reconstruct a significant amount of confidential data. To find out how realistic this threat was, the agency conducted a wargaming exercise. John Abowd, the Census Bureau’s chief scientist and a driving force behind its new privacy strategy, described the results as “conclusive, indisputable, and alarming.”
In a court filing last April, Abowd wrote that “our simulated attack showed that a conservative attack scenario using just 6 billion of the over 150 billion statistics released in 2010 would allow an attacker to accurately re-identify at least 52 million 2010 Census respondents (17% of the population) and the attacker would have a high degree of confidence in their results with minimal additional verification or field work.”
That does sound alarming! A lot of commentators have taken Abowd’s characterization at face value, treating it as an established fact that the 2010 privacy approach was inadequate and a new strategy was needed. But Abowd’s summary of the experiment was misleading.
A 62 percent error rate
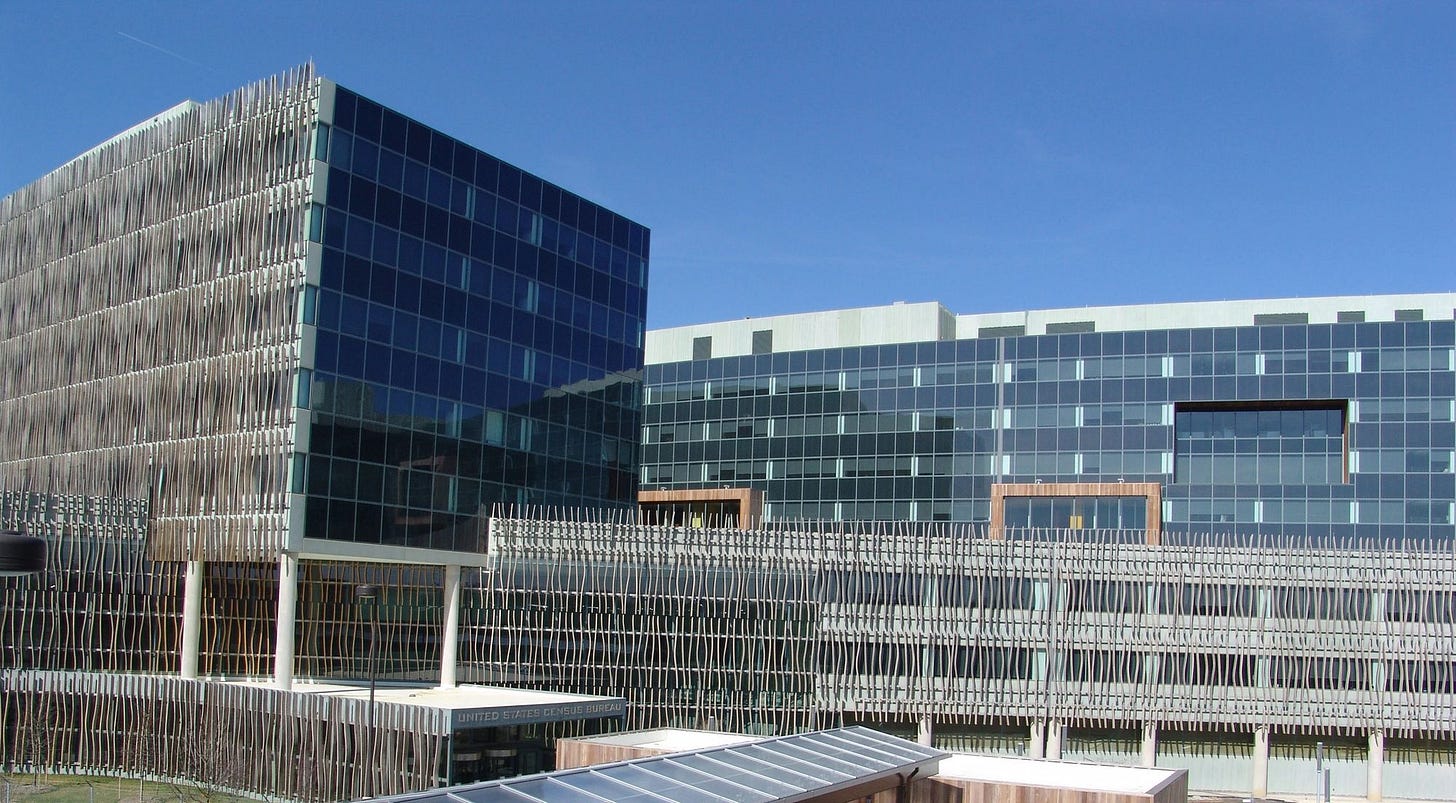
The experiment started with summary statistics like “this block had 13 non-Hispanic white people” or “this block had 37 people under the age of 18.” Using commercial linear programming software, the Census Bureau produced what amounted to a giant spreadsheet listing age, sex, race, and ethnicity for all 308 million people who were in the United States in 2010. For example, a row might indicate that Block 1004 of Census Tract 11 had a 42-year old non-hispanic white man.
However, most rows in the reconstructed data—54 percent—didn’t match anybody in the real world. The data also didn’t include anyone’s name or address. To make it useful, it needed to be re-identified. So the Census Bureau cross-referenced it with commercial marketing databases, looking for matches based on age and sex.
When the researchers did this, they found that 62 percent of re-identified records were wrong. Either the name and address in the commercial database didn’t match the underlying census record, or else the reconstructed data had the wrong race or ethnicity.
And without access to confidential census data, a hypothetical private party wouldn’t have an easy way to determine which reconstructed records were the correct ones. They’d just be stuck with a database that was only 38 percent accurate.
Census Bureau officials have repeatedly portrayed this experiment as demonstrating the real-world threat of re-identification attacks. For example, Michael Hawes, a senior advisor for privacy, has described the reconstructed data as “highly accurate” despite the fact that a majority of records were not, in fact, accurate.
Earlier I quoted the Census Bureau’s John Abowd arguing that an attacker could have a “high degree of confidence in their results with minimal additional verification or field work.” The “field work” he has in mind seems to be contacting millions of people to ask for their race and ethnicity. But that’s the same work you’d have to do if you were constructing the database from scratch. So it’s not clear how this reconstruction technique would be useful to someone trying to obtain confidential census data.
This debate isn’t over. The Census Bureau told me that it is preparing to release additional details about its reconstruction experiments. These details may demonstrate that particular populations are at heightened risk of re-identification. But the data the agency has released so far isn’t very convincing.
A culture clash
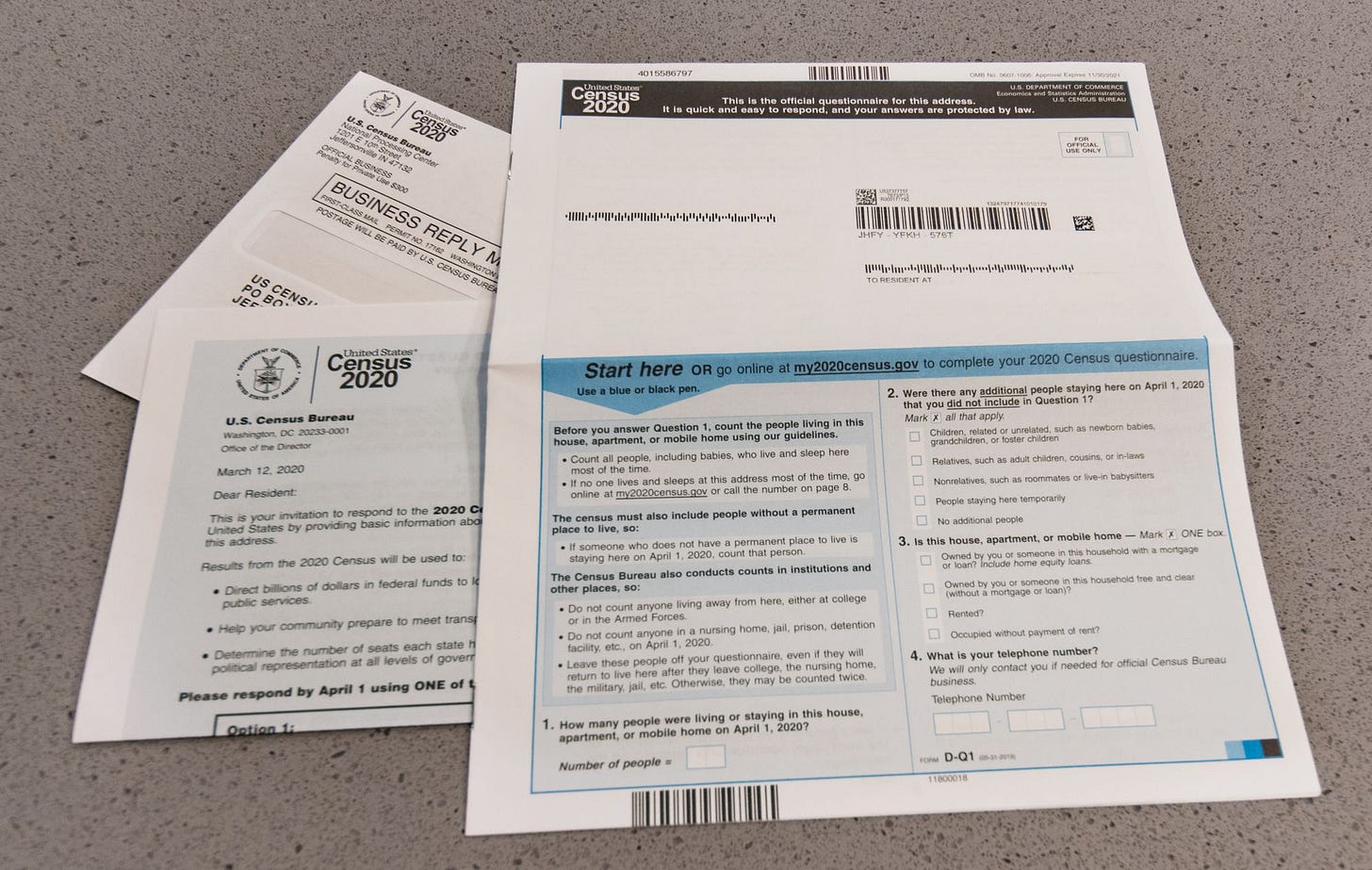
Privacy advocates like Abowd believe it’s a mistake to fixate on the practicalities of any specific re-identification strategy. They believe the Census Bureau’s experiment demonstrated that Census data was vulnerable to a database reconstruction attack—at least in theory—and that it is only a matter of time before someone figures out a practical way to exploit the vulnerability.
And I think this is where you see a major cleavage between privacy advocates (including a lot of computer scientists who study privacy) and social scientists. The first group views privacy as paramount and sees the usefulness of census data as a secondary concern. They favor proactively guarding privacy even against theoretical re-identification threats that haven’t been demonstrated or even invented yet.
“Privacy research is littered with people who were pretty sure they understood the risk and turned out to be wrong,” said computer scientist Frank McSherry in a recent phone interview. “If you release information and then realize that was too much, you can't call it back.”
The gold standard for privacy advocates is a methodology called differential privacy. It was co-invented by McSherry and serves as the foundation for the Census Bureau’s new privacy scheme. Differential privacy offers a mathematically rigorous way to quantify how much privacy is lost when new information is released.
The framework includes a parameter called epsilon that lets a data publisher fine-tune the tradeoff between privacy and utility. A higher epsilon means more accurate data but a greater risk of exposing an individual response.
But critics argue that differential privacy is too rigid for applications like the decennial census.
“The definition and measure of privacy embedded in Differential Privacy is poorly matched to actual risk of disclosure,” wrote Jane Bambauer in a legal brief last year. “Because privacy is defined in a manner that is insensitive to context, including which types of data are most vulnerable to attack, Differential Privacy compels data producers to make bad and unnecessary tradeoffs between utility and privacy. Re-identification attacks that are much more feasible, and thus much more likely to occur, are treated exactly the same as absurdly unlikely attacks.”
In a sense, this is why privacy advocates like differential privacy. They believe no one can predict which attacks are “absurdly unlikely,” so the only safe course of action is to protect against every conceivable attack. That might dramatically reduce the usefulness of the published data, but privacy advocates care about this much less than social scientists do.
Ruggles, the demographer, has been trying for years to convince privacy advocates that differential privacy isn’t a good fit for census data. In 2019, he traveled to the University of California at Berkeley to meet with several prominent experts on differential privacy.
“They were very nice,” Ruggles said. “But they didn't believe me. They said, ‘you know we just have to do this. It will be all right.’”
“They seemed kind of arrogant,” he added. “Particularly since they can't document a single case where anybody has ever been identified from the census.”
The practical threat isn’t clear
The question I kept coming back to in conversations with computer scientists and the Census Bureau was: what would a real-world privacy harm look like?
McSherry suggested a scenario involving banks or insurance companies using reconstructed data to discriminate on the basis of racial or ethnic categories. An organization could do this even if it only had the kind of noisy, probabilistic data produced by the Census’s reconstruction attack.
But discrimination on the basis of race or ethnicity is illegal, so it seems unlikely that a bank or insurance company would launch a formal program to do this. More importantly, it’s not obvious that noisy data about race is helpful to someone who wants to discriminate on the basis of race. Pretty good proxies for race are readily available.
For example, many cities in the United States are racially segregated. Certain blocks are overwhelmingly white, while others are predominantly black. An organization that wanted to discriminate against black people could assume that everyone on a majority-black block is black. This strategy wouldn’t be perfect, but it could easily be more effective than using the noisy data from the Census Bureau’s reconstruction attack.
Felten, the computer scientist, argues that public perception is a major concern for the Census Bureau. If people don’t trust the confidentiality of census data, response rates will fall and future census data will be less accurate.
I think that’s a valid point, but once again I struggle to translate this abstract concern into a concrete threat. Obviously, if someone hacked Census Bureau servers and obtained scanned PDFs of peoples’ actual census forms, that would damage public trust in the Census Bureau. But a reconstructed database with a 38 percent accuracy rate isn’t going to move the needle
Even if computer scientists dramatically improved on the Census Bureau’s methodology in the next few years, enabling them to predict someone’s race with 60 or 80 or 90 percent certainty, I’m still not sure people would care. These would still just be guesses, and anyone who considered their race a sensitive subject would retain plausible deniability.
The Census Bureau, for its part, argues that it has no alternative. The law prohibits publishing data from which any individual’s responses “can be identified.” The agency argues that its database reconstruction experiment proved that individual responses can, in fact, be identified from data published in 2010.
I’m not so sure. Someone who produces a reconstructed database that’s 38 percent accurate hasn’t identified anyone’s census responses—they’re just guessing.
The threat of re-identification seems to be most serious for the smallest blocks. For blocks with fewer than 10 people, the Census Bureau found that its re-identified matches were correct 72 percent of the time. And the agency estimates that using better commercial data could have pushed this as high as 96 percent. That really is worrying.
But there’s a simple solution to this that even skeptics like Ruggles support: get rid of the smallest blocks. For the 2030 Census, the Census Bureau can and should merge the 1-house block at the start of this story with one of its neighbors. They should do the same thing for other blocks with single-digit populations. This would make it much harder to pinpoint their individual responses—without needing to add any fake people to census results.