I can't stop thinking about the implications of ChatGPT
Predictions are hard, especially about AI.
Like a lot of other people, I’ve spent the last couple of weeks playing around with OpenAI’s chatbot, ChatGPT. And I’ve been totally blown away.
I’m not alone, of course. More than a million people started using ChatGPT in the first few days it was publicly available, and my Twitter timeline has been filled with people posting screenshots of their favorite conversations.
ChatGPT is remarkably versatile. It can explain obscure philosophical doctrines, debug computer code, write and revise recipes, and compose stories on any topic—and in any style—the user requests. It remembers context from one question to the next, so if its initial answer isn’t what the user is looking for, the user can ask ChatGPT, in plain English, to revise it.
ChatGPT has gotten so much attention in recent days that I’m going to assume you are familiar with the basics. If not, I encourage you to read Sam Hammond or Maxim Lott or Jon Stokes. In this post I want to speculate on how this technology might impact the American economy in the coming years and decades.
The Hype Cycle
As a reporter I’ve always tried to be early in identifying and understanding technologies that will have a big economic impact. One thing I’ve learned over the last 15 years is that it’s difficult!
Take self-driving cars as an example. I wrote my first article on the topic in 2008. At that time I was excited about the technology but thought it was still decades from commercialization.
But then the technology advanced faster than I expected, and by 2017 I—like a lot of people—though we were just a few years away from large-scale commercialization. So when I took a job at Ars Technica that year, I made self-driving cars a major part of my beat. Since then, however, progress has seemed slower. Today I’m still optimistic about the technology’s long-term prospects but I’m unsure if it will be commercially significant before the end of the decade.
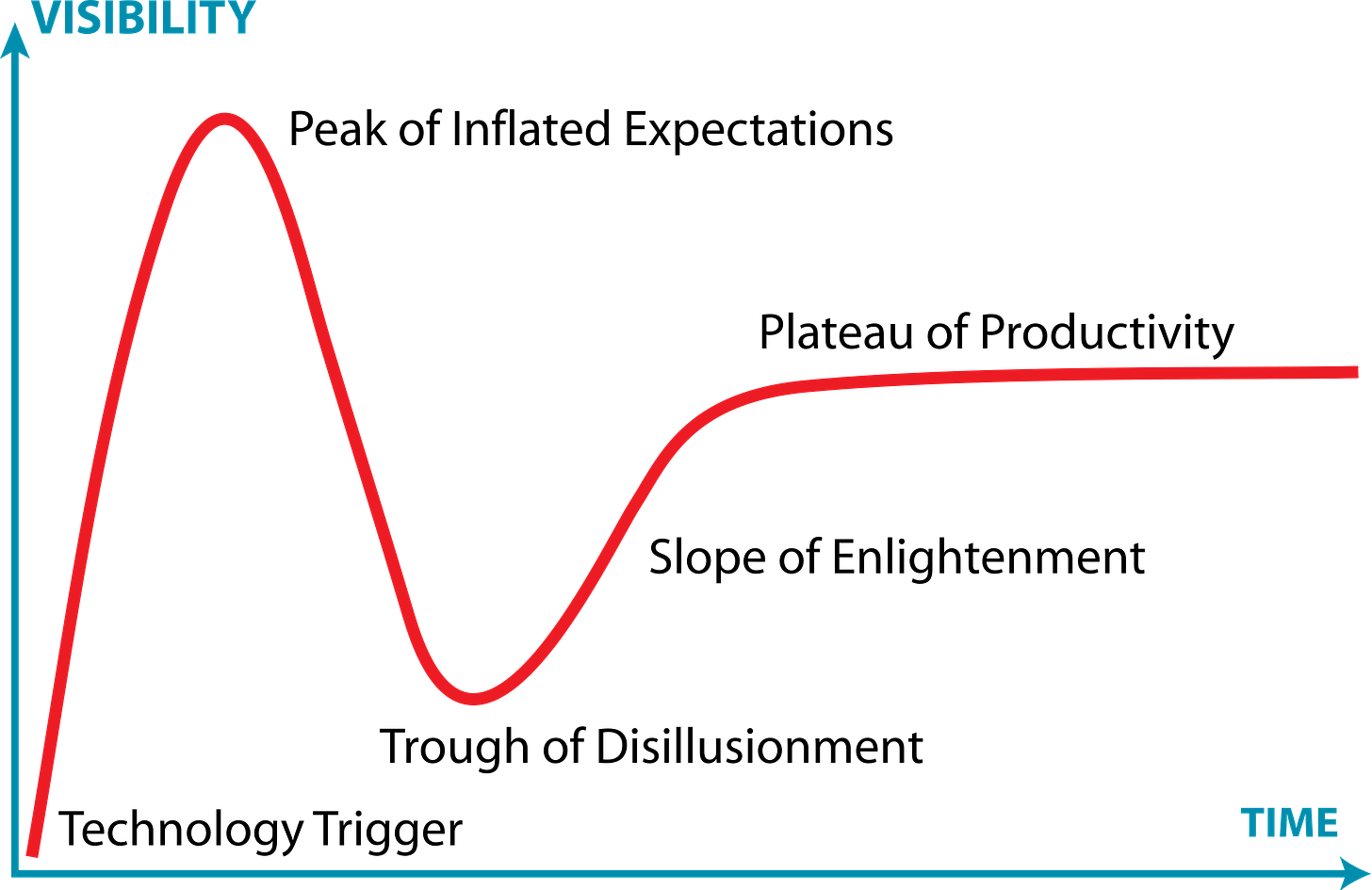
This pattern is so common that the technology consulting firm Gartner developed a fun model of it called the Gartner Hype Cycle. Self-driving cars reached the Peak of Inflated Expectations around 2018, and we’re now somewhere around the Trough of Disillusionment. With luck, we’ll ascend the Slope of Enlightenment and reach the Plateau of Productivity—where self-driving cars are a normal, boring part of the economy—within a decade or two.
Generative AI systems like ChatGPT and Stable Diffusion are still on that first upward slope—a lot of people are just starting to take this technology seriously, and our minds are starting to run wild with the possibilities.
Right now this technology feels as promising as any new technology I’ve written about over the last 20 years. My early optimism about self-driving cars was tempered by the fact that it seemed to still be decades away from commercialization. In contrast, programmers are already using GitHub Copilot, a variant of OpenAI’s GPT technology, to help them write computer code.
In this respect, the release of ChatGPT feels more like Steve Jobs’s 2007 introduction of the iPhone. The iPhone’s value was immediately clear to anyone who watched his January 2007 presentation. The iPhone quickly set the standard for the smartphone industry, attracting imitators and creating a huge new market for mobile apps.
I think something similar is likely to happen with ChatGPT. Big tech companies like Google and Amazon will scramble to match its capabilities, and a bunch of new startups will be created to apply generative AI to fields like law, accounting, graphic design, public relations, tutoring, and so forth.
And then I’m not sure what will happen next. Maybe a lot of these companies will bump up against the limits of the technology and fail, just as a lot of early self-driving startups failed. Or maybe as with smartphones, the technology will work fine and everything will be up and to the right. Right now I can talk myself into either scenario.
Could ChatGPT do my job?
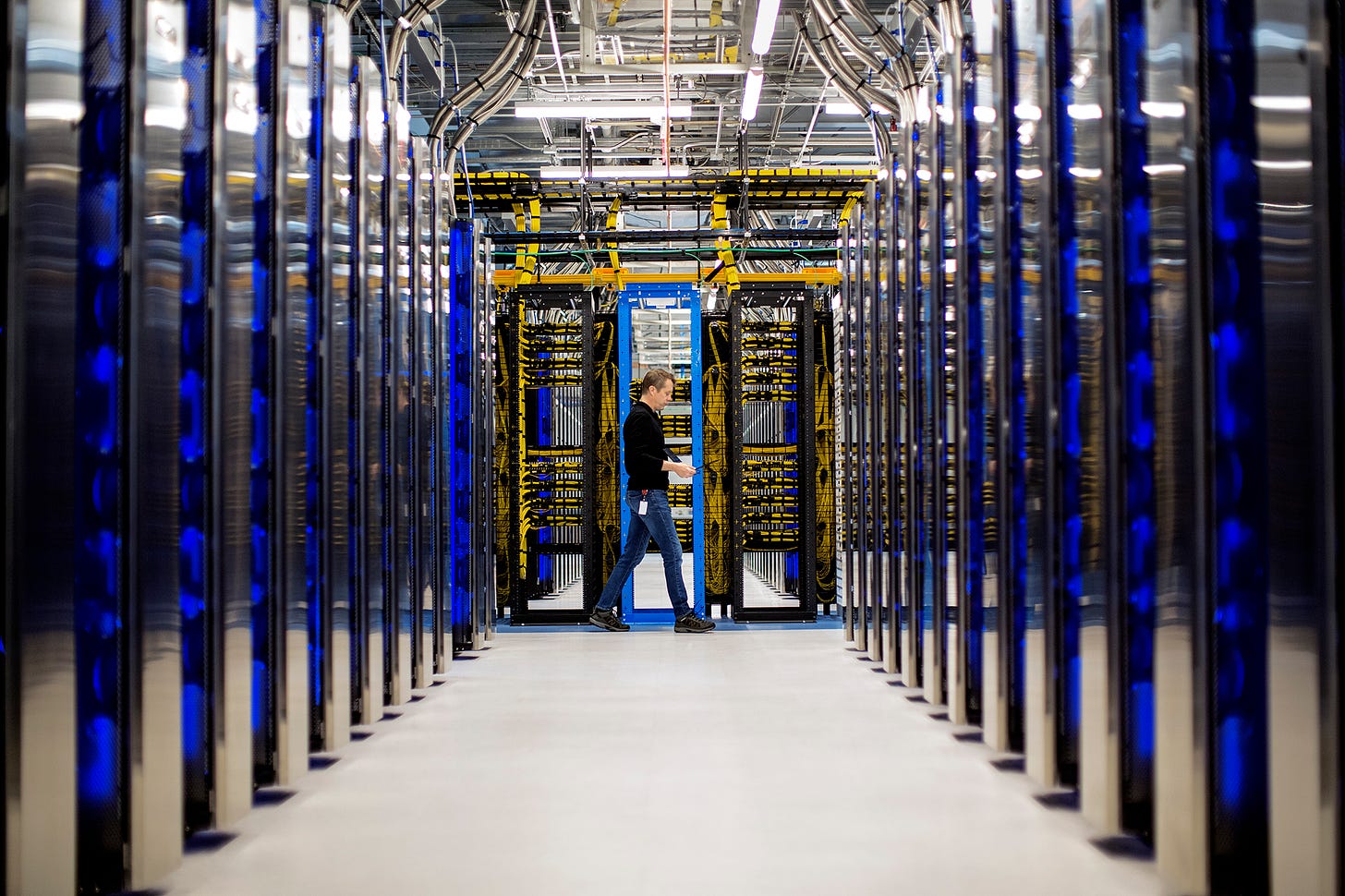
One way to get a handle on the possibilities is to imagine applying this technology to the industry I know best—journalism.
A few days ago I asked ChatGPT to write an article about last month’s collapse of FTX. I supplied ChatGPT with a few key facts about the collapse. The resulting article was surprisingly good. It was formatted like a news article, had perfect grammar, and pulled in relevant context I didn’t supply in my prompt. For example, ChatGPT accurately noted that Alameda Research was “a major liquidity provider” for FTX.
At the same time, ChatGPT’s piece was far from being the most interesting or insightful thing I’ve read on the topic. It didn’t break any news or offer any new insights. And that’s not surprising because ChatGPT was trained on data that only runs through the end of 2021. It literally didn’t know anything about FTX’s collapse other than what I told it.
Of course, anyone trying to make an AI-driven news site would fix that. They’d have software to scrape press releases, other news sites, blogs, and social media in real time. That could allow the AI to produce timely articles with much richer detail.
If I’m being honest, a significant share of the articles I wrote for Ars Technica—maybe 20 or 30 percent by article count—didn’t do all that much more than summarize documents that were publicly available online. For example, every quarter Tesla would report how many cars it produced during the preceding quarter, and I’d write a 400-word news article basically summarizing the announcement and providing readers with historical context. Usually I would add a few sentences of my own analysis and predictions, but I’m not confident that a suitably trained version of GPT couldn’t do that equally well—or that readers would have missed those parts of the stories if I’d left them out.
For my more ambitious Ars stories, I’d often interview experts, policy advocates, or industry insiders to try to better understand the topic I was writing about. ChatGPT can’t talk on the phone, but it could certainly do text-based chats with experts. So could a computer program do my job?
For what it’s worth, ChatGPT itself is skeptical of this idea. “Using ChatGPT to conduct interviews with sources and incorporate what it learns into a news article would likely result in a poorly written and poorly structured article that does not accurately represent the information provided by the source,” the chatbot told me.
I would like to think this is true! It’s nice to think that my best work does more than just summarize information provided to me by other people—that I synthesize the information and offer original insights in ways that a computer program couldn’t replicate. After using ChatGPT for a few days, I still think that’s probably true, at least for the current iteration of the technology. Will it still be true in five or ten years? It’s hard to say.
Even if humans maintain their monopoly on in-depth analysis, this technology could still have a big impact on the organization of newsrooms. In many digital newsrooms, writers are expected to write a mix of short, newsy articles and more in-depth pieces. Maybe in a decade they’ll delegate the shorter articles to an AI (probably fact-checked by a human reporter) and free up human reporters to do more in-depth reporting.
Rethinking the newsroom
It’s also very possible that instead of replacing human reporters, AI software could work with human reporters and make them more productive.
A fair amount of my time as a reporter is spent writing and re-writing sentences, paragraphs, and even whole sections in an effort to find exactly the right words. There’s also a fair amount of busywork looking up things like titles and affiliations, dates, stock prices, and so forth.
Generative AI has the potential to automate a lot of this work. A decade from now a reporter’s workflow might look something like this:
Write a bullet list of the facts that should be in an article, along with some hints about tone and structure,
Have a generative AI draft an article (or maybe 5 or 10 candidate articles) based on this prompt, and
Read through the article(s), make notes of the things you want to change, and return to step one to refine the prompt.
This is similar to the process that programmers have gone through for decades: write source code, use a compiler to turn the source code into an executable program, and then run the program to see if it works.
This is also how AI art gets created today: an artist writes a text prompt, has the AI generate a batch of images, and then sees which ones they like best and repeats the whole process.
I could see this kind of workflow dramatically improving the productivity of reporters, especially in the (fairly common) case where an article has a few sentences of new information followed by a bunch of background summarizing earlier developments in the story. The AI should already know all the background information and be able to fill it in automatically. The reporter would just have to skim the finished article to make sure the AI didn’t make any mistakes.
But why stop there? Once you have software that can automate the drafting of news articles, a lot of new opportunities open up. For example, there are many events—an election, a baseball game, a hurricane—that unfold in realtime, with new information coming in every few minutes. Traditional news organizations only generate a new story every few hours, but a generative AI could generate new stories continuously. In 10 years you might be able to visit ESPN.com at any time and read a news story about any in-progress baseball game that includes events that happened just a few seconds earlier.
An AI could also generate different stories for different readers:
Someone who reads a lot about a topic (say the war in Ukraine) could get an article that focuses on the latest developments, while a newbie could get an explainer with a lot of background information.
If a member of Congress comments on the war in Ukraine, the software could include her quote in the version of the story read by her constituents.
If a reader has ties to a particular part of Ukraine, stories could provide more detail about how the war is unfolding in that area.
Obviously this is all easier said than done, and it’s hard to predict exactly how the industry will evolve. But the important point is that generative AI is going to open up a lot of new options for how to organize a newsroom. Some might involve replacing human reporters with computer programs, while others might involve using software to enhance the productivity of human reporters. Most likely we’ll get some of both, in a complex and unpredictable mixture.
How generative AI can fail
One of the most interesting responses I got from ChatGPT was this one: I asked it to give me directions from my Washington DC neighborhood to Dulles Airport in Northern Virginia. And if you just glance at the answer it seems about right, listing approximately the right streets in approximately the right order.
But if you pull up a map and actually trace out the route suggested by ChatGPT, you realize that it’s total nonsense. In step two it says to turn left when it should say right. In step three it says to turn right when it should say left. These directions would be useless for someone who actually needed to drive to the airport.
Traditional software is good at solving complex but well-defined problems like “give me directions between these two points on this map,” but hopeless at understanding subjective human concepts. ChatGPT turns this on its head: it’s surprisingly good at grasping the nuances of human speech, but it fails at straightforward computational tasks like finding the shortest route between two points on a map.
Under the hood, ChatGPT uses a fantastically complex statistical model to estimate the likelihood that sequences of words are associated with other sequences of words. This allows it to understand seemingly complex relationships like “Paul Krugman is more neoliberal than Bernie Sanders” or “Alameda Labs was a major liquidity provider for FTX.” But it leaves it helpless when trying to answer questions that require a non-trivial amount of non-verbal reasoning.
For example, just now I asked ChatGPT to give me a prime number between 5,343,532,432 and 5,353,532,432, and it cheerfully responded with 5,353,523,421. This is not a prime number! For starters, it is divisible by 3.
ChatGPT is smart enough to know that 5,353,523,421 is between the other two numbers, which honestly is pretty impressive in its own right. But ChatGPT has no idea what a prime number actually is. (Weirdly, ChatGPT does know how to generate a computer program to determine whether a number is prime. So it seems like this should be a fixable problem.)
Some critics of this technology like to put a lot of stress on this point, arguing that ChatGPT doesn’t “really” understanding what it’s saying and is merely engaging in a sophisticated form of pattern matching. That’s not wrong exactly, but I think it underplays how often human beings do the same thing. From time to time, we all have to reason about abstract concepts without fully understanding the underlying reality—for me this happens every time I take my car into the shop, for example.
The difference is that we have a sophisticated understanding of which tasks humans can do reliably and which they can’t, and human societies have developed habits and institutions to accommodate the limits of human cognition. We don’t let a 7-year-old drive a car, for example, and we don’t let someone perform surgery unless they’ve received appropriate training.
In contrast, we’re just starting to learn about the capabilities and limitations of generative AI. And as we learn more, we might realize that the limitations are much more significant than we thought at the outset.
Again, I think self-driving cars are a helpful point of comparison. It was relatively easy for computer scientists to build a car that could drive 99 percent of trips correctly. This led to a wave of initial euphoria in the mid-2010s. However, self-driving cars ultimately need to drive as safely as human beings, and that’s actually a very high bar: about one fatal crash every 100 million miles. Reaching that level of safety—and then proving that they’ve reached it—is going to be a huge challenge.
We might face similar challenges applying technology like ChatGPT to new industries. Nobody would want to use an AI tax preparer that does their taxes wrong five percent of the time. So until the software surpasses human levels of accuracy, I expect generative AI to create jobs more than it destroys them. Because you’ll still need human beings to figure out what questions to ask the AI and then check that the answers are correct.
In the short run, I think this might worsen the “average is over” problem that Tyler Cowen wrote about a decade ago. Generative AI is likely to increase the productivity of the most knowledgeable and talented people in creative industries, which could allow them to claim a larger share of the work and leave their less talented peers with worse job prospects.
It’s hard to say what might happen in the longer run. Maybe enough white-collar jobs will get automated that the college wage premium will decline and labor will shift to more hands-on jobs from welding to nursing. Or conversely, maybe there will be a bunch of new AI-related jobs and highly educated workers will continue to do much better than everyone else. Right now it’s way too early to predict.